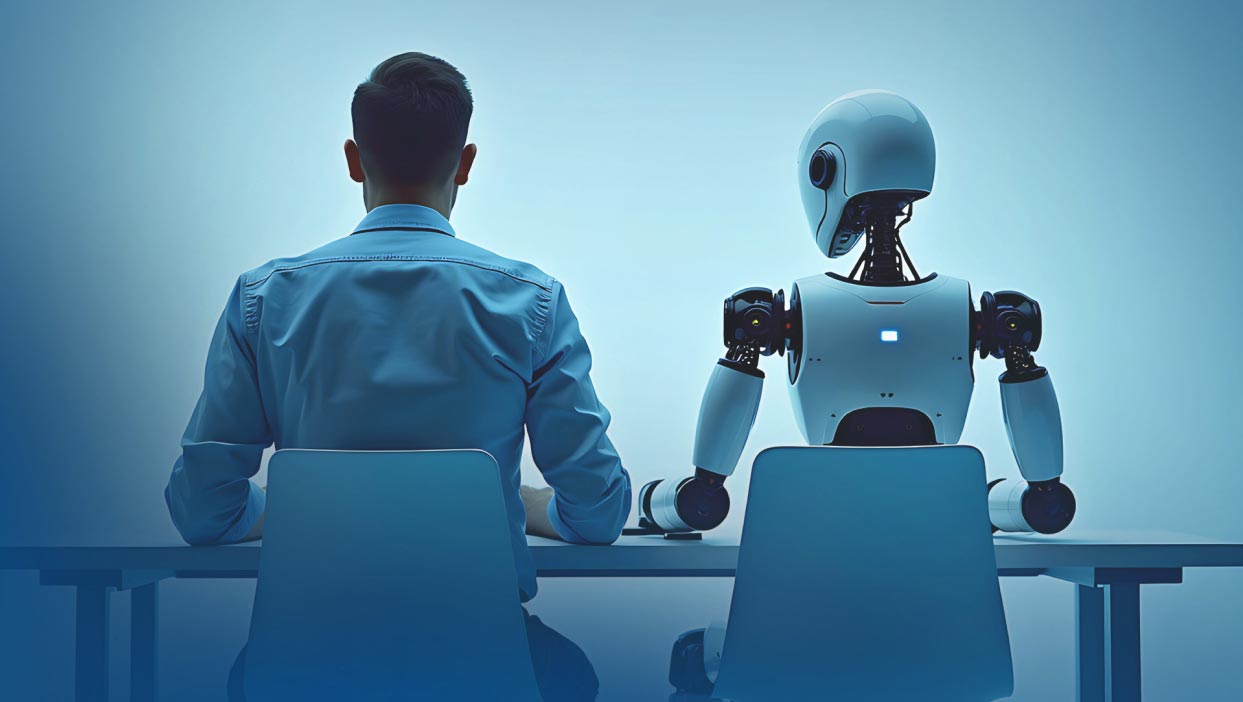
Generative AI in Cybersecurity
Opportunities and Challenges in AI-Driven Threat Detection.
Authenticity matters in business and relationships, and the noticeable lack of authenticity in AI-generated communications can limit its practical application.
Generative AI in cybersecurity is a perfect example of the dichotomy between automation and human intelligence – AI can provide all the best data, but you have to be careful about how you use that data to inform your decisions.
I think about authenticity a lot in my role at DirectDefense, because to put it bluntly, the disconnect that can occur from an artificial engagement versus an authentic one can fairly quickly translate to a degradation of service quality.
The trick is in the balance of information and action.
Anyone who’s been in the information security space for a while understands that artificial intelligence and machine learning can give you access to a ton of great information. For example, we often use phone number spoofing and reconnaissance of corporate databases to impersonate an employee and gain access to accounts and information via a company’s help desk. This type of employee information is easy to get and exploit in this way.
Read More: Using Social Engineering to Conduct Physical and Wireless Penetration Testing
The help desk employees we’ve bypassed with convincing spoofs and fakes have lacked the training and awareness necessary to question these types of communications; further, our success demonstrates the believability of generative AI.
However, there’s a prevailing opinion that AI could never replace human intelligence or human context. But what exactly do we mean by “human intelligence” and “human context” – and how do we balance them with AI?
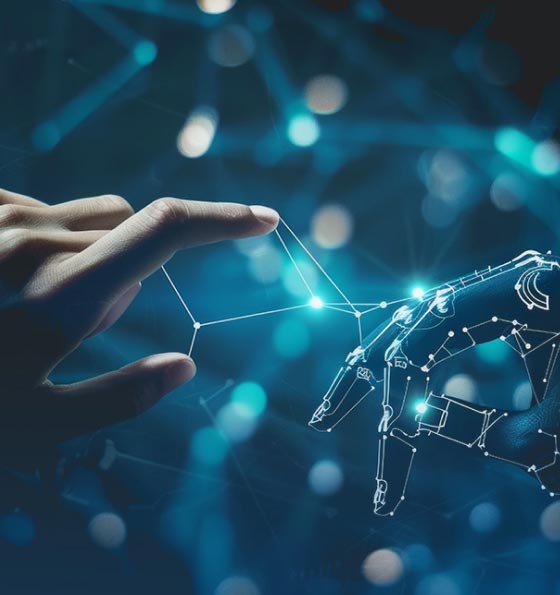
Defining AI vs. Human Intelligence in Cybersecurity
It’s fairly easy to tell when something has been produced by AI. It goes back to authenticity – you can make the best AI model in the world, but it can’t read between the lines. Content can seem very robotic and will lack the unique voice of the writer.
AI will spit out what you want based on clear and concise directions, but the presentation of information will be missing the “so what” factor. If we hand AI-generated security monitoring data over to our clients with no explanation, we wouldn’t be giving them any context to act upon.
Only human intelligence and human context can take that monitoring data and interpret it to filter out how much of the network activity is automated attacks and what might be targeted attacks by an individual or group.
90% of attacks are crimes of opportunity. Phishing emails and robo calls are automated attacks that test for weak defenses – similar to someone walking down a street checking to see which cars are unlocked instead of just breaking a car window to get inside.
And now, generative AI enables more realistic and believable attacks by regionalizing email messages and imitating people’s voices on phone calls and voicemails.
Read More: Why Does Bob From HR Need Your Email Password? Because Bob’s Not Bob.
The same AI tools we’re using in cybersecurity are available to attackers, allowing them to get more creative. The best thing we can do is continue implementing ways to combat them – something AI cannot do on its own.
A security operations center (SOC) is a great example of how to leverage AI alongside human intelligence and human context to effectively monitor cybersecurity.
The major function of a SOC is investigation and validation – using AI to collect data and generate reporting. What’s missing in most SOCs and services is the next level of authenticity that recognizes companies are run by real people with real needs and concerns. AI models can spit out the data but simply cannot tap into those customer nuances – that’s where humans can intervene to interpret the data and make best-fit recommendations to meet a company’s unique needs and goals.
Many of the cybersecurity models we use today have some type of AI-generated input and output – and we have to approach our use of these models with an advisor mindset. A data analyst will put information in and take information out, but an advisor looks at the intent of the information, what is really going on inside the network, and what it means for the customer.
Box-Checking vs. Nuance in Cybersecurity Compliance
Cybersecurity compliance is another great example of the intersection of AI and human input. Compliance is all about following rules and guidelines – checking boxes to say, ‘yes, we have X and Y, but no, we don’t have Z’.
While generative AI can provide an analysis of what a client’s cyber program has or doesn’t have relative to compliance requirements, it will lack important nuance.
Let’s use a car as an example:
Compliance requires four wheels. An AI model conducts an assessment and reports that yes, the car has four wheels. What that AI model didn’t pick up on is the fact that one of those wheels is a donut.
In reality, the car can’t go more than a handful of miles and can’t drive over a certain speed. But only human context can identify that reality and recommend a new tire be installed.
In a security context, a client’s business can be less secure if AI doesn’t understand the nuances of why something was done. Our clients aren’t trying to make themselves less secure – our job is to gain an understanding of the situation and use that context to drive better decision-making.
People historically don’t love compliance, but approaching it with understanding and sound advice can make it a lot easier.
The Power of AI, Downstream Impacts, and Where It’s Headed
The biggest concern I have with AI is that people aren’t fully aware of what it’s capable of. Attackers are getting more creative with how they’re using generative AI, and employees are often fairly easy to fool because there is a lack of awareness of how advanced it can be.
We’re entering a new era in cybersecurity where we’re learning how to respond to AI-generated attacks by using AI.
I’ve worked in cybersecurity for a long time and was discussing the power of AI in this space back in 2012 in the context of The Turing Test. This test, proposed by Alan Turing in 1949, is used to judge a machine’s ability to imitate human behavior in a way that’s indistinguishable from human behavior.
AI has arguably passed the Turing Test at this point in time. Generative-AI capabilities across communications are successfully mimicking human behavior and executing successful scams to break into networks and steal sensitive data.
We may be at an inflection point with AI where it has major capabilities but is also posing threats that are yet to be fully understood and managed. Cybercriminals are always looking for something to exploit, and as MSSPs, we’re constantly helping clients bridge the gap between attacker capabilities and cybersecurity.
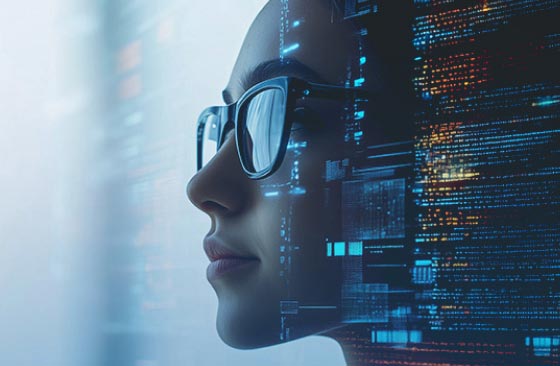
We may be at an inflection point with AI where it has major capabilities but is also posing threats that are yet to be fully understood and managed. Cybercriminals are always looking for something to exploit, and as MSSPs, we’re constantly helping clients bridge the gap between attacker capabilities and cybersecurity.
The biggest impacts are employee security awareness training and education, and a security program that applies human intelligence to provide context around the nuances of a specific client’s business that AI simply can’t do.
Managing AI in cybersecurity really comes down to authenticity and human connections, and making sure we don’t overlook the point that you can’t substitute a human connection, understanding, and lived experiences with language models.
At the same time, language models can provide better information than any normal human can – but they can’t contextualize it into human experience.
Wondering if your cybersecurity program has enough authenticity? Talk to us about blending AI with human intelligence to safeguard your business and stay one step ahead of generative AI.